How do we know where the economy is heading today?
What is the present situation of the economy, and in which direction is it heading? What is the present rate of economic growth? National accounts and other economic statistics offer information on the economic situation, but they are published with a considerable delay. Information on the GDP of the present quarter does not arrive until several months later. Even so, many economic decisions require the support of real-time information. Short-term forecast models, so-called nowcasting models, enable evaluation of the state of the economy before official statistics are completed.
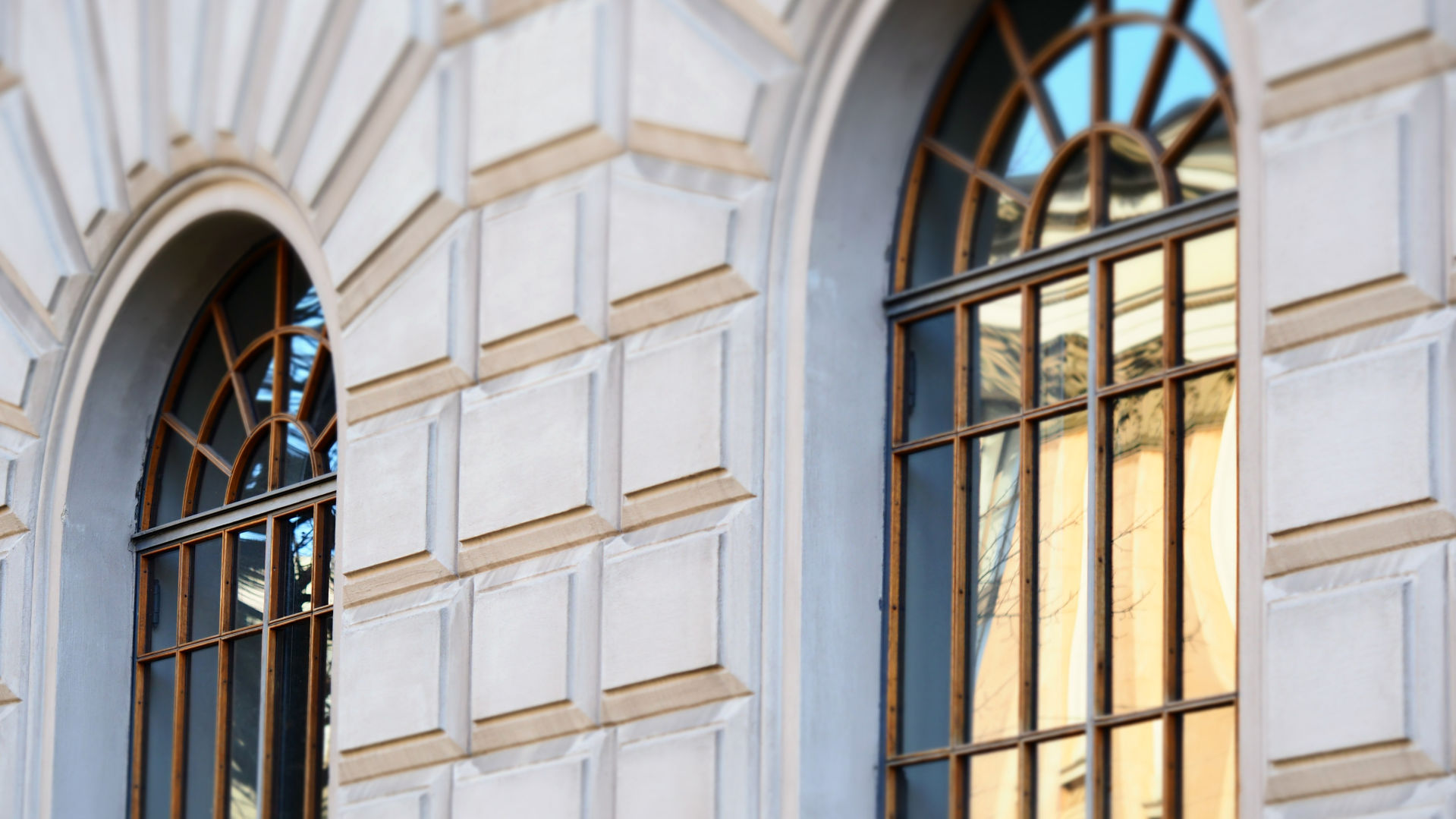
What are nowcasting models used for?
Central banks need real-time information on the state of the economy and the direction of development in order to support their decisions. An informed view on economic development and related factors is required to maintain price stability. Monetary policy measures affect the economy with a considerable delay, emphasising the importance of a monetary policy that is formulated to meet future developments.
Short-term forecasts also serve as a basis for longer-term forecasts. Twice a year, the Bank of Finland publishes its macroeconomic forecast for the Finnish economy, where the forecast period is 2–3 years. An overall picture of the state of the economy formed by nowcasting models and the most recent indicators is used for determining the starting point for the forecast.
Forecasts are also important to many other economic agents. Economic decisions are often based on expectations about the future. Economic policy-makers need to know the present state of the economy so that financial and monetary policy can be designed to fit the prevailing cyclical conditions.
Economic policy’s capacity to balance cyclical fluctuations requires that turnarounds be detected as early as possible, so that necessary measures can be taken in good time. Slow reaction may deepen recessions unnecessarily and, in the worst case, an ill-timed cyclical policy may even strengthen economic volatility. Early recognition of cyclical turning points is one of the most important, and at the same time most challenging, tasks in economic forecasting.
Correspondingly, corporate investments and recruitments are long-term commitments, whose profitability often depends on how the economy develops in the future. Consumers can also utilise economic forecasts when contemplating big decisions, such as house purchases.
What do nowcasting models forecast?
The most closely monitored indicator of the current macroeconomic situation is probably the quarterly calculated gross domestic product, GDP. It indicates the volume of products and services produced by the economy, and its growth rate is reflected in the development of many other core economic performance figures, such as unemployment and purchasing power.
Typically, nowcasting models are used for forecasting GDP, or more specifically the change in the seasonally adjusted GDP volume relative to the previous quarter. In this connection, short term (nowcasting) refers to the forecasting of the GDP of the present or recently completed quarter.
The first information on quarterly GDP is published no sooner than two months after the end of each quarter. In addition, the first GDP figure must also be adjusted several times, when new information on economic events is accumulated.
The recent history of the economy must also be "forecasted". As there is a delay in the completion of statistics, depending on the juncture no official statistics are available on the previous 2–5 months. Before the official statistics are published, the view on economic growth inevitably relies on forecasts.
Big picture most significant in GDP forecasts
Also in short-term forecasting, attention is typically paid to the trend of economic growth. The trend describes development after the impact on the economy of very temporary contingencies has been filtered away.
In a small economy such as Finland, quarterly changes in GDP may fluctuate a lot from one quarter to the next. One tenth of one per cent of the Finnish GDP equals an income of only EUR 50 million, so sporadic business transactions may swing the growth rate of individual quarters considerably.
A trend adjusted for contingencies better describes the development lines and cyclical conditions of the economy. Nowcasting models are also better in forecasting the trend.
A study of the growth rate of the Finnish GDP in 1990–2015 shows how the seasonally adjusted growth rate fluctuates around the short-term trend (Chart 1). The growth rate may fluctuate considerably from one quarter to the next, but the development of the trend provides a pretty good general picture of the economic growth in recent decades.
During the recession in the 1990s, the economy contracted for a relatively long period. In the middle of the decade, economic growth accelerated and continued at a brisk pace up to the financial crisis in 2008. Only the IT bubble in the early 2000s slightly slowed down the growth rate. The recession in the real economy that followed the financial crisis depressed GDP rapidly and deeply. In 2010–2011 the Finnish economy recovered for a while, but soon it took a downward turn. Growth edged slightly into the black in 2015.
In 1990–2015, quarterly GDP grew by 0.4% on average. Thus the annual growth rate was about 1.6%. The weak growth in recent years has slowed the average rate.
Nowcasting models in the Bank of Finland
The Bank of Finland uses several different models for short-term economic forecasting. Practical experience has demonstrated that the best picture of the present economic situation is obtained through different versatile approaches.
As a rule, nowcasting models use monthly statistics published with a short delay, such as confidence indicators, turnover data of different industries and share prices. For example, confidence indicators, which describe consumers' and companies' opinions of the economy, forecast short-term economic developments fairly well. The indicators are based on a similarly repeated survey, whose results for the present month are published before the end of the month.
The indicator modelJuho Kostiainen, Seppo Orjasniemi and Jukka Railavo (2013) Lyhyen aikavälin ennustemalli Suomen kokonaistuotannolle ('Nowcasting model for total Finnish output'). BoF Online 3/2013. developed at the Bank of Finland is based on a so-called factor-augmented vector autoregressive model, which utilises a very large group of economic indicators. The advantage of the model is its capacity to use various sources of information and from them filter out an up-to-date picture of the economic state and direction. The latest version of the Bank of Finland indicator model uses information on the economic situation from 76 different statistical series.
The weakness of the model is the typical non-transparency of factor models, because the breakdown of factors affecting the forecasts is challenging. In addition, the model is exposed to different structural interruptions that often appear in indicator series and may cause systematic forecast errors.
So-called bridge models are statistical models based on individual indicator series. Due to their simplicity and stability, they probably are the most frequently used models for short-term forecasting. A bridge model explains quarterly changes in GDP using values from a monthly published indicator series.
The confidence indicator for the overall economy compiled by the European Commission, connecting the consumer and corporate confidence indicators, fairly well describes the quarterly changes in GDP (Chart 3). Bridge models can be applied using many different indicator series, and the most typical is to monitor an average forecast based on a large group of indicators.
Assessment of forecasting ability
Assessment of the forecasting ability of models forms an important part of the use and development of forecasting models. Comparison of performed forecasts and actual outcomes is a natural way of assessing the forecasting accuracy of models. The forecasting error is the difference between forecast and actual outcome.
Firstly, a good forecasting model should be unbiased, which means that the model does not systematically generate higher or lower forecasts than the outcomes. Lack of bias can be analysed by calculating the mean forecast error (MFE) for a certain period. The MFE for an unbiased forecasting model does not significantly deviate from zero.
Lack of bias alone is not enough, as the model should also be accurate. An accurate model generates forecasts that come close to the actual outcomes. The root mean square forecast error (RMSFE) is a frequently used forecast error measure, which is obtained by computing the mean of squared forecast errors and extracting the square root of that. The closer the RMSFE figure is to zero, the more accurate is the forecasting model. In relative terms, the measure gives greater weight to large forecast errors.
When realised forecast errors are assessed and new models developed, it is of vital importance to bear in mind what information was available at the time when the forecast was made. With hindsight, it is easy to build models that explain positive developments, when the variables to be forecasted are already known for a long period. However, in practice, this often gives an overly optimistic picture of the model's forecasting ability. The challenge of the economic forecaster is to assess development in a situation when future observations are not yet known.
To avoid second-guessing, it is always good to use pseudo out-of-sample forecasts in assessment of forecasting ability. Thus the forecast is made only on the basis of the part of statistical data that was available prior to the period to be forecasted. In that case, the forecasting models are compared in the same situation as the one when the forecaster applied the model.
GDP a moving target for the forecaster
The information on GDP development provided by national accounts is updated in connection with new statistical releases. The statistical information becomes more detailed as the statistical authority gets access to more information on the development of different sectors of the economy. A more accurate picture of GDP is obtained through personal tax information, for example, but this information is not final before the tax forms have been filed and the taxation decisions have been made.
Thus the first information on GDP is not final. In this respect, GDP is a moving target, which complicates the forecaster's task. Even if the forecast were to exactly hit the growth rate in the statistical release, it is very possible that the forecast will be off when the statistics get updated. From a practical point of view, this means that perfect accuracy cannot be expected from nowcasting forecasts.
The uncertainty connected with the actual statistics can be assessed by studying the difference between data in the first releases and the latest information.The latest quarterly national accounts statistics considered in this study were released on 16 March 2016. It should also be noted that statistical principles are revised regularly, which for its part has changed historical growth rates. At times, the adjustment of GDP growth rates has been considerable (Chart 4). In particular, the exceptionally large drop in the first quarter of 2009 was not yet revealed in the first statistical release.
The adjustment of statistics can be assessed by using the same measures as in the study of forecast errors. In 2008–2015, the average statistical change of quarterly GDP growth was 0.2%. The figure is comparable with the MFE figure presented above. Thus the growth rates in the first statistical releases have been slightly higher than the latest growth rates, i.e. the picture of GDP growth has slightly deteriorated as the data have been adjusted.
The deviation of statistical changes in growth rates has been fairly wide. In half of the cases, the statistical change was larger than –0.3–0.5%. Correspondingly, 75% of the changes fitted into the –0.5–0.8% interval. The standard deviation of statistical changes was 0.99. The figure is comparable with the RMSFE figure measuring the forecast error of the forecasting models.
The standard deviation of statistical changes can also be considered as a sort of lower limit of forecast errors of forecasting models, and better accuracy cannot be expected. Even if the forecasting model were always to hit exactly the figure of the first statistical release, as the statistics are updated the RMSFE figure of the forecasting model in question would increase to close to one in a comparison with the latest statistical GDP release.
A study of statistical changes reminds us that, in the interpretation of growth rates, it is important to take the uncertainty of the statistics themselves into account. The same applies to growth rate forecasts. Since contingencies affecting economic development are difficult to forecast in practice, it is more meaningful to relate the forecasts to the development of the GDP trend. Not even the best forecasting models recognise all fluctuations around the trend. Nevertheless, the forecasting models quite accurately follow the lines laid down by the trend (Chart 5).
Which forecasting model to use?
As a rule, the more up-to-date information there is available to the forecasting models, the more accurate forecasts can they generate. Different models perform differently, however, depending on the forecast horizon. If, for example, the purpose is to forecast the second quarter growth rate in early June, which forecasting model should be selected?
Below follows a comparison of the forecasting accuracy of different models at different points of time relative to the quarter to be forecasted (Chart 6). The study concerns forecasting model RMSFE figures for forecast errors in 2008–2015 relative to the point of time for computing the forecast.
The study shows that at the beginning of June, 30 days prior to the end of the quarter to be forecasted, an indicator model based on dynamic factors has performed best (Chart 6). Measured by the RMSFE figure, it has generated smaller forecast errors than alternative models.
However, the indicator model seems to perform worse when the forecast horizon is longer. Before the start of the quarter to be forecasted (t-90 days), the most accurate forecasts have been generated with bridge models based on confidence indicators. A slightly better result can be obtained with bridge models when a mean forecast by bridge models computed with several different confidence indicators is used. In this study (Chart 6), the mean forecast of bridge models is based on a forecast using nine different confidence indicators.
GDP development can also be forecasted using autoregressive models, which are simple statistical time series models based on earlier GDP development. Typically, autoregressive models are used as a benchmark relative to the forecasting ability of more complex models. The study shows that indicator-based models perform significantly better than autoregressive models (Chart 6).
In its simplest form, an autoregressive model can be formed by assuming that the growth rate of the next quarter will be the same as in the previous quarter. A slightly more advanced autoregressive model is obtained by assuming that the growth rate linearly depends on previous observations and the long-term mean value. Very early forecasts cannot be generated with autoregressive models, because forecasts based on a change from the previous quarter are not available until a month before the end of the quarter to be forecasted.
Statistics Finland's trend indicator on output offers early information on monthly developments of value added and it is based on the same sources of information as the actual quarterly national accounts. Its forecasting ability is at its best after the second monthly statistical release of the quarter.
A bridge model forecast based on the trend indicator on output for the previous quarter does not deviate much from an autoregressive model forecast. This result is expected, because the trend indicator does not offer, to any appreciable extent, additional information on economic development compared to the quarterly national accounts.The trend indicator on output is retrospectively reconciled with the quarterly national accounts. In assessments of its forecasting ability, it is particularly important to use historical releases, as has been done in this study.
Approximately 45 days after the end of the quarter, Statistics Finland publishes a flash estimate based on the trend indicator on output, which predicts the final GDP growth rate comparatively well. Two months after the end of the quarter, the first actual release of statistical information is published, and after that no better picture can be generated with short-term forecasting models.
Present economic situation
What do the short-term models say about the present economic situation? Below, economic development is examined with the help of the above-described forecasting models in the light of the most recent indicator data available.
The Bank of Finland indicator model predicted a growth of 0.4% for first-quarter GDP compared with the previous quarter.Computed on the basis of data released by 16 May 2016. The result hit the same figure as the flash estimate published by Statistics Finland. For the second quarter, the model predicts growth of 0.0%. Thus the model predicts that the relatively rapid growth in early 2016 was temporary.
A bridge model based on the overall economic confidence indicator predicts 0.2% growth for the second quarter. Correspondingly, the consumer confidence indicator, which in principle has correlated fairly well with economic development, forecasted 0.1% growth. The industrial output expectations, in turn, predict a 0.1% contraction. The construction, service and retail confidence indicators predict slightly steeper growth, but in historical terms their forecasting ability has been considerably weaker.
Foreign business cycle indicators can also be used in Finnish economic forecasting. International cyclical fluctuations have typically also been reflected in Finland, so foreign indicators describe our domestic developments well. The IFO and ZEW indices, the euro area confidence indicator, and the euro area and US Purchasing Manager Indices on average predict around 0.4% growth in the Finnish economy in the second quarter. However, in recent years Finland has lagged behind international economic development, so these indicators have systematically generated overly optimistic forecasts for Finland.
Overall, indicator data predict sluggish but positive growth for the second quarter. However, growth will probably remain below the long-term average.
How should forecasts be interpreted?
Given the considerable uncertainty associated with forecasting and the deviating forecast figures provided by different forecasting models, interpretation of short-term forecasts may be challenging. At least three things should be considered in the interpretation of forecasts.
Firstly, quarterly GDP figures are affected by many contingencies, the forecasting of which is very difficult. Therefore there are no grounds to assume that an individual forecast would be correct with an accuracy of one decimal point. The forecast figure should be considered as an expected value within a wider forecast range. An individual forecast figure, a so-called point estimate, indicates the most plausible outcome on the basis of the indicator material and forecasting model. This does not rule out the possibility that alternative outcomes close to the point estimate are relatively probable as well.
Secondly, it is more reasonable to interpret forecast figures as indicators of an economic trend, i.e. of a development adjusted for contingencies. Thus it seems conceivable that a forecast is good if the realised growth figures keep close to the general trend and the forecast figure indicates the prevailing cyclical position of the economy. When a forecast focuses on the trend, it avoids attaching too much importance to diverging observations and contingencies, which after all reveal very little about the direction of economic development.
Thirdly, a study of different forecasting models reminds us that extensive indicator material and different modelling approaches should be used impartially in the assessment of the cyclical position. Far too often, the public debate focuses on an individual economic performance figure, from which very far-reaching conclusions may be drawn on the outlook for the whole national economy. The user of a forecast may without noticing cherry pick among forecast figures the ones that correspond with his or her own preconceptions.
The problem of selection is illustrated by bridge model forecasts generated on the basis of 24 different indicators (Chart 7). If you look for it, it is easy to find an indicator generating a forecast that temporarily corresponds with your own preconception. But overall the collection of different indicators has followed the trend fairly well.
Generating a thorough snapshot of the situation requires a comprehensive and balanced economic analysis, which in various ways observes different sources of information, carefully assesses the uncertainty related to them and paints a consequent overall picture on the basis of them. Correctly interpreted short-term model forecasts offer decision-makers valuable information on economic development.